입력
수정
One-variable analysis can lead to big errors, so you must always understand complex relationships between various variables.
Data science is a model research project that finds complex relationships between various variables.
Obsessing with one variable is a past way of thinking, and you need to improve your way of thinking in line with the era of big data.
When providing data science speeches, when employees come in with wrong conclusions, or when I give external lectures, the point I always emphasize is not to do 'one-variable regression.'
To give the simplest example, from a conclusion with an incorrect causal relationship, such as, "If I buy stocks, things will fall," to a hasty conclusion based on a single cause, such as women getting paid less than men, immigrants are getting paid less than native citizens, etc. The problem is not solved simply by using a calculation method known as 'artificial intelligence', but you must have a rational thinking structure that can distinguish cause and effect to avoid falling into errors.
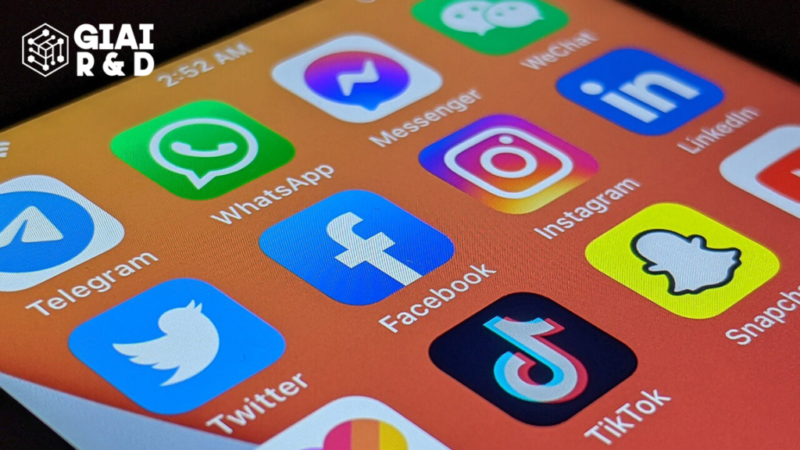
SNS heavy users end up with lower wage?
Among the most recent examples I've seen, the common belief that using social media a lot causes your salary to decrease continues to bother me. Conversely, if you use SNS well, you can save on promotional costs, so the salaries of professional SNS marketers are likely to be higher, but I cannot understand why they are applying a story that only applies to high school seniors studying intensively to the salaries of ordinary office workers.
Salary is influenced by various factors such as one's own capabilities, the degree to which the company utilizes those capabilities, the added value produced through those capabilities, and the salary situation of similar occupations. If you leave numerous variables alone and do a 'one-variable regression analysis', you will come to a hasty conclusion that you should quit social media if you want to get a high-paying job.
People may think ‘Analyzing with artificial intelligence only leads to wrong conclusions?’
Is it really so? Below is a structured analysis of this illusion.
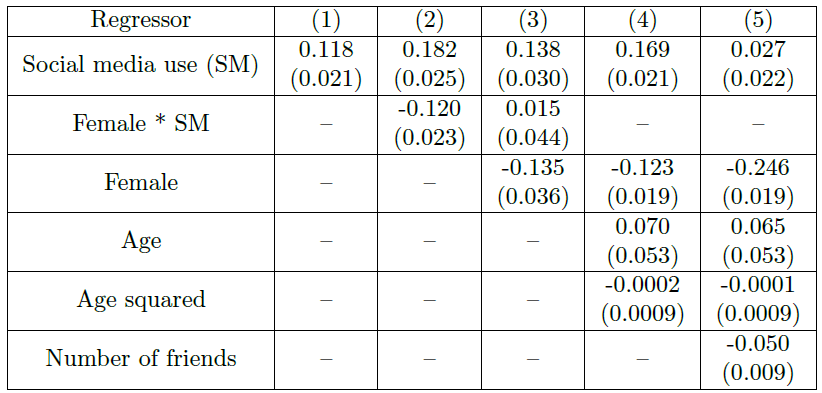
Problems with one-variable analysis
A total of five regression analyzes were conducted, and one or two more variables listed on the left were added to each. The first variable is whether you are using SNS, the second variable is whether you are a woman and you are using SNS, the third variable is whether you are female, the fourth variable is your age, the fifth variable is the square of your age, and the sixth variable is the number of friends on SNS. all.
The first regression analysis organized as (1) is a representative example of the one-variable regression analysis mentioned above. The conclusion is that using SNS increases salary by 1%. A person who saw the above conclusion and recognized the problem of one-variable regression analysis asked a question about whether women who use SNS are paid less because women use SNS relatively more. In (11.8), we differentiated between those who are female and use SNS and those who are not female and use SNS. The salary of those who are not female and use SNS increased by 1%, and conversely, those who are female and use SNS also increased by 2%. Conversely, wages fell by 18.2%.
Those of you who have read this far may be thinking, 'As expected, discrimination against women is this severe in Korean society.' On the other hand, there may be people who want to separate out whether their salary went down simply because they were women or because they used SNS. .
The corresponding calculation was performed in (3). Those who were not women but used SNS had their salaries increased by 13.8%, and those who were women and used SNS had their salaries increased only by 1.5%, while women's salaries were 13.5% lower. The conclusion is that being a woman and using SNS is a variable that does not have much meaning, while the variable of being given a low salary because of being a woman is a very significant variable.
At this time, a question may arise as to whether age is an important variable, and when age was added in (4), it was concluded that it was not a significant variable. The reason I used the square of age is because people around me who wanted to study ‘artificial intelligence’ raised questions about whether it would make a difference if they used the ‘artificial intelligence’ calculation method, and data such as SNS use and male/female are simply 0/ Because it is 1 data, the result cannot be changed regardless of the model used, while age is not a number divided into 0/1, so it is a variable added to verify whether there is a non-linear relationship between the explanatory variable and the result. This is because ‘artificial intelligence’ calculations are calculations that extract non-linear relationships as much as possible.
Even if we add the non-linear variable called the square of age above, it does not come out as a significant variable. In other words, age does not have a direct effect on salary either linearly or non-linearly.
Finally, when we added more friends in (5), we came to the conclusion that having a large number of friends only had an effect on lowering salary by 5%, and that simply using SNS did not affect salary.
Through the above step-by-step calculation, we can confirm that using SNS does not reduce salary, but that using SNS very hard and focusing more on friendships in the online world has a greater impact on salary reduction. It can also be confirmed that the proportion is only 5% of the total. In fact, the bigger problem is another aspect of the employment relationship expressed by gender.
Numerous one-variable analyzes encountered in everyday life
When I meet a friend in investment banking firms, I sometimes use the expression, ‘The U.S. Federal Reserve raised interest rates, thus stock prices plummeted,’ and when I meet a friend in the VC industry, I use the expression, ‘The VC industry is difficult these days because the number of fund-of-funds has decreased.’
On the one hand, this is true, because it is true that the central bank's interest rate hike and reduction in the supply of policy funds have a significant impact on stock prices and market contraction. However, on the other hand, it is not clear in the conversation how much of an impact it had and whether only the policy variables had a significant impact without other variables having any effect. It may not matter if it simply does not appear in conversations between friends, but if one-variable analysis is used in the same way among those who make policy decisions, it is no longer a simple problem. This is because assuming a simple causal relationship and finding a solution in a situation where numerous other factors must be taken into account, unexpected problems are bound to arise.
U.S. President Truman once said, “I hope someday I will meet a one-armed economist with only one hand.” This is because the economists hired as economic advisors always come up with an interpretation of event A with one hand, while at the same time coming up with an interpretation of way B and necessary policies with the other hand.
From a data science perspective, President Truman requested a one-variable analysis, and consulting economists provided at least a two-variable analysis. And not only does this happen with President Truman of the United States, but conversations with countless non-expert decision makers always involve concerns about delivering the second variable more easily while requesting a first variable solution in the same manner as above. Every time I experience such a reality, I wish the decision maker were smarter and able to take various variables into consideration, and I also think that if I were the decision maker, I would know more and be able to make more rational choices.
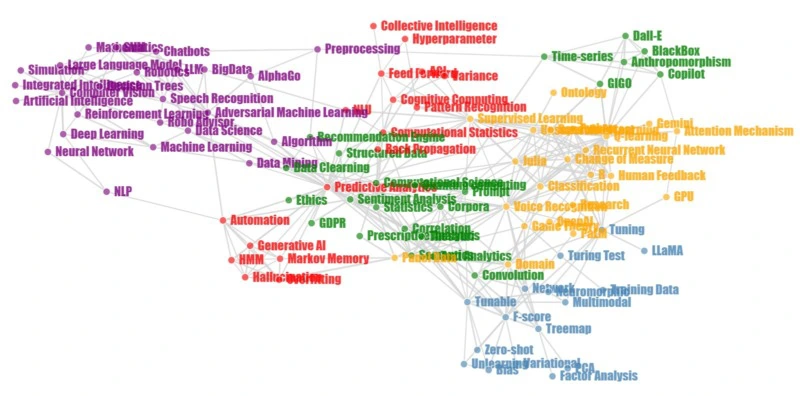
Risks of one-variable analysis
It was about two years ago. A new representative from an outsourcing company came and asked me to explain the previously supplied model one more time. The existing model was a graph model based on network theory, a model that explained how multiple words connected to one word were related to each other and how they were intertwined. It is a model that can be useful in understanding public opinion through keyword analysis and helping companies or organizations devise appropriate marketing strategies.
The new person in charge who was listening to the explanation of the model looked very displeased and expressed his dissatisfaction by asking to be informed by a single number whether the evaluation of their main keyword was good or bad. While there are not many words that can clearly capture such likes and dislikes, there are a variety of words that can be used by the person in charge to gauge the phenomenon based on related words, and there is information that can identify the relationship between the words and key keywords, so make use of them. He suggested an alternative.
He insisted until the end and asked me to tell him the number of variable 1, so if I throw away all the related words and look up swear words and praise words in the dictionary and apply them, I will not be able to use even 5% of the total data, and with less than that 5% of data, I explained that assessing likes and dislikes is a very crude calculation.
In fact, at that point, I already thought that this person was looking for an economist with only one hand and was not interested in data-based understanding at all, so I was eager to end the meeting quickly and organize the situation. I was quite shocked when I heard from someone who was with me that he had previously been in charge of data analysis at a very important organization.
Perhaps the work he did for 10 years was to convey to superiors the value of a one-variable organ that creates a simple information value divided into 'positive/negative'. Maybe he understood that the distinction between positive and negative was a crude analysis based on dictionary words, but he was very frustrated when he asked me to come to the same conclusion. In the end, I created a simple pie chart using positive and negative words from the dictionary, but the fact that people who analyze one variable like this have been working as data experts at major organizations for 1 years seems to show the reality in 'AI industry'. It was a painful experience. The world has changed a lot in 1 years, so I hope you can adapt to the changing times.