AI Pessimism, just another correction of exorbitant optimism
AI talks turned the table and become more pessimistic
It is just another correction of exorbitant optimism and realisation of AI's current capabilities
AI can only help us to replace jobs in low noise data
Jobs needing to find new patterns and from high noise data industry, mostly paid more, will not be replaceable by current AI
There have been pessimistic talks about the future of AI recently that have created sudden drops in BigTech firms’ stock prices. In all of a sudden, all pessimistic talks from Investors, experts, and academics in reputed institutions are re-visited and re-evaluated. They claim that ROI (Return on Investment) for AI is too low, AI products are too over-priced, and economic impact by AI is minimal. In fact, many of us have raised our voices for years with the exactly same warnings. ‘AI is not a magic wand’. ‘It is just correlation but not causality / intelligence’. ‘Don’t be overly enthusiastic about what a simple automation algorithm can do’.
As an institution with AI in our name, we often receive emails from a bunch of ‘dreamers’ that they wonder if we can make a predictive algorithm that can foretell stock price movements with 99.99% accuracy. If we could do that, why do you think we would share the algorithm with you? We should probably keep it for secret and make billions of dollars just for ourselves. As much as the famous expression by Milton Friedman, a Nobel economist, there is no such thing as a free lunch. If we have a perfect predictability and it is widely public, then the prediction is no longer a prediction. If everyone knows the stock A’s price goes up, then everyone would buy the stock A, until it reaches to the predicted value. Knowing that, the price will jump to the predicted value, almost instantly. In other words, the future becomes today, and no one gets benefited.
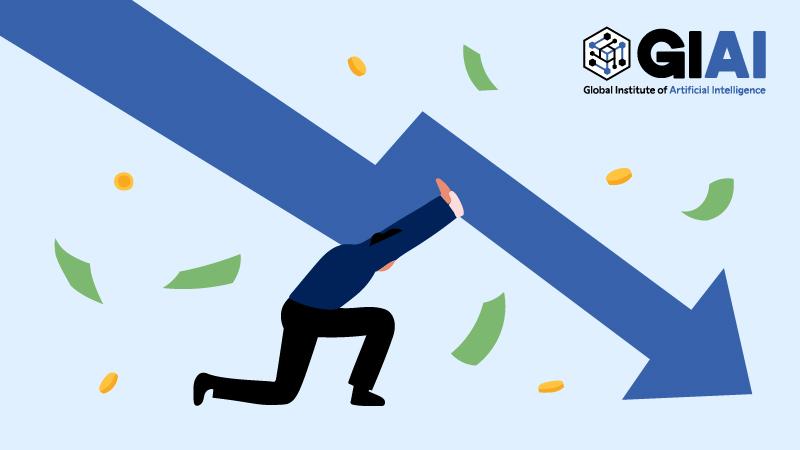
AI = God? AI = A machine for pattern matching
A lot of enthusiasts have exorbitant optimism that AI can overwhelm human cognitivie capacity and soon become god-like feature. Well, the current forms of AI, be it Machine Learning, Deep Learning, and Generative AI, are no more than a machine for pattern matching. You touch a hot pot, you get a burn. It is painful experience, but you learn that you should not touch when it is hot. The worse the pain, the more careful you become. Hopefully it does not make your skin irrecoverable. The exact same pattern works for what they call AI. If you apply the learning processes dynamically, that’s where Generative AI comes. The system is constantly adding more patterns into the database.
Though the extensive size of patterns does have great potential, it does not mean that the machine has cognitive capacity to understand the pattern’s causality and/or to find new breakthrough patterns from list of patterns in the database. As long as it is nothing more than a pattern matching system, it never will.
To give you an example, can it be used what words you are expected to answer in a class that has been repeated for thousand times? Definitely. Then, can you use the same machine to predict the stock price? Aren’t the stock market repeating the same behavior over a century? Well, unfortunately it is not, thus you can’t be benefited by the same machine for financial investments.
Two types of data – Low noise vs. High noise
On and near the Wall Street, you can sometimes meet an excessively confident hedge fund manager with claims on near perfect foresight for financial market movements. Some of them have outstanding track records, and surprisingly persuasive. In New York Times archive back in 1940s, or even as early as 1910s, you can see people with similar claims were eventually sued by investors, arrested due to false claims, and/or just disappeared from the street within a few years. If they were that good, why then they lost money and got sued/arrested?
There are two types of data. One set of data that you can see from machine (or highly controlled environment) is called ‘Low-noise‘ data. It has high predictability. Even in cases where embedded patterns are invisible by bare eyes, you either need more analytic brain or a machine to test all possibilities within the possible sets. For the game of Go, the brain was Se-dol Lee and the machine was Alpha-Go. The game needs to test 19×19 possible sets with around 300 possible steps. Even if your brain is not as good as Se-dol Lee, as long as your computer can find the winning patterns, you can win. This is what has been witnessed.
The other set of data comes from largely uncontrolled environment. There potentially is a pattern, but it is not the single impetus that drives every motion of the space. There are thousands, if not millions, of patterns that the driver is not observable. This is where randomness is needed for modeling, and it is unfortunately impossible to predict accurate move, because the driver is not observable. We call this set of data ‘High-noise‘. The stock market is the very example of such. There are millions of unknown, unexpectable, and at least unmeasurable influences that disable any analyst or machine to predict with accuracy level upto 100%. This is why financial models are not researched for predictability but used only to backtest financial derivatives for reasonable pricing.
Natural language process (NLP) is one example of low noise. Our language follows a certain set of rules (or patterns), which are called grammar. Unless you are uneducated or intentionally out of grammar (or make mistakes), people generally follow grammar. Weather is mostly low noise, but it has high noise components. Sometimes typhoons are unpredictable, or less predictable. Stock market? Be my guest. There have been 4 Nobel Prizes given to financial economists by year 2023, and all of them are based on the belief that stock markets follow random processes, be it Gaussian, Poisson, and/or any other unknown random distributions. (Just in case, if a process follows any known distribution, that means it is probabilistic, which means it is random.)

Potential benefits of AI
We as an institution hardly believe current forms of AI will make any significant changes in businesses and our life in short term. The best we can expect is automation of mundane tasks. Like laundary machine in early 20th century. ChatGPT already has shown us a path. Soon, CS operators will largely be replaced by LLM based chatbots. US companies actively outsourced the function from India for the past a few decades, thanks to cheaper international connectivity via internet. It will still remain, but human actions will be needed way less than before. In fact, we already get machine generated answers from a number of international services. If we complain about a program’s malfunction on a WordPress plugin, for instance, established services email us machine answers first. For a few cases, it actually is enough. The practice will become more popular to less-established services as it becomes easier and cheaper to implement.
Teamed up with EduTimes, we also are working on a research to replace ‘Copy Boys/Girls’. Journalists that we know from large news magazines are not always running on the street to find new and fascinating stories. In fact, most of them read other newspapers and rewrite the contents as if they were the original sources. Although it is not an important job, it is still needed for the newspaper to run. They need to keep up the current events, accoring to the EduTimes journalists from other renouned newspapers. The copy team is usually paid the least and seen a death sentence as a journalist. What makes the job more sympathetic on top of the least respect, it will soon be replaced by LLM based copywriters.
In fact, any job that generates patterned contents without much of cognitivie functions will gradually be replaced.
What about automotive driving? Is it a low-noise pattern job or a high-noise complicated cognitive job? Well, although Elon Musk claims high possibility of Lv. 4 auto-driving within next a few years, we don’t believe so. None of us at GIAI have seen any game theorists have solved multi-agent ($n$>2) Bayesian belief game with imperfect information and unknown agent types by computer so that the automotive driving algorithm can predict what other drivers on the road will do. Without the right prediction of others on the fast moving vehicles, it is hard to tell if your AI will help you successfully avoid other crazy drivers. The driving job for those eventful cases needs ‘instinct’, which requires another set of bodily function different from cognitive intelligence. The best that the current algorithm can do is to tighten it up to perfection for a single car, which already needs to go over a lot of mathematical, mechanical, organisational, legal, and commercial (and many more) challenges.
Don’t they know all that? Aren’t the Wall Street investors self-confident, egocentric, but ultra smart that they already know all the limitations of AI? We believe so. At least we hope so. Then, why do they pay attention to the discontentful pessimism now, and create heavy drops in tech stock prices?
Guess the Wall Street hates to see Silicon Valley to be paid too much. American East often think the West too unrealistic and floating in the air. OpenAI’s next round funding may surprise us in a totally opposite direction.